🎓Victor COULON thesis defense
Wednesday 29 January 2025 at 14h00
Phd Thesis JCA room, CERFACS, Toulouse
Turbulent combustion of lean hydrogen/air premixed flames: from numerical assessment of Lewis number effects to deep learning modeling for large eddy simulations
ED MEGEP
https://youtube.com/live/Z2whppkYO64?feature=share
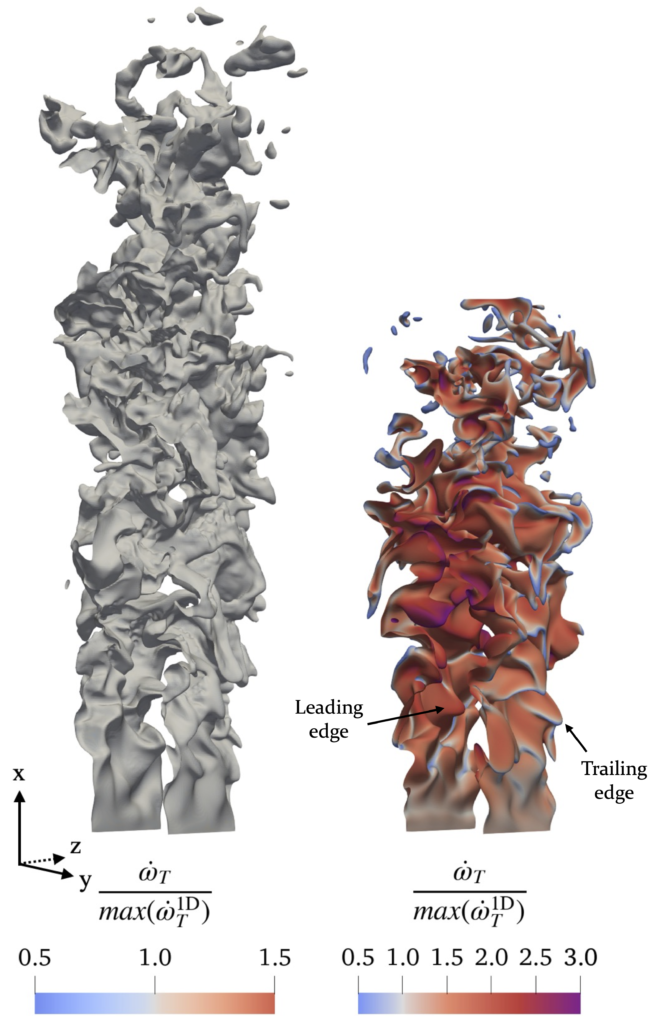
With the current need of transitioning away from fossil fuels, hydrogen is considered a promising alternative. However, lean hydrogen/air premixed flames can exhibit synergistic effects between turbulence and intrinsic instabilities, leading to drastic flame acceleration and posing challenges for numerical simulations. These effects are neglected in current state-of-the-art subgrid-scale (SGS) models for Large Eddy Simulation (LES) and still require development, as they are governed by diffusive phenomena beyond the resolution of LES meshes. Meanwhile, the advent of deep learning (DL) offers new opportunities for combustion modeling, where high-fidelity data can be harnessed to explore new LES modeling strategies. The first part of this work presents a physical analysis of a turbulent lean hydrogen/air premixed flame under atmospheric conditions. Three-dimensional Direct Numerical Simulations (DNS) are performed using the AVBP solver to assess Lewis number effects on a thermo-diffusively unstable jet flame compared to a stoichiometric methane/air flame, both sharing the same unstretched laminar flame properties and turbulent flow conditions. The analysis reveals a strong impact of stretch effects on the hydrogen flame structure, resulting in substantial local deviations from the corresponding unstretched laminar flamelet gradients and burning rates. In particular, the preferential diffusion of hydrogen relative to heat induces local variations in equivalence ratio in curved flame regions and sensitivity to strain effects in flat flame regions, causing the lean hydrogen flame to consume reactants twice as fast as the methane flame. Moreover, one-dimensional stagnation counterflow flamelets are shown to fairly estimate the hydrogen burning rates observed in the DNS, despite their simplification with only strain rates normal to the flame direction. In the second part, this work explores new modeling strategies using deep learning (DL) to develop data-driven SGS models for LES. An ad-hoc filtering methodology is proposed to elaborate a training database from instantaneous DNS snapshots of the flame configuration discussed in the first part. Then, a Convolutional Neural Network (CNN) is parametrized within a supervised learning framework to develop two data-driven SGS models based on filtered hydrogen reaction terms. First, an efficiency model dedicated to the Thickened Flame formalism for LES (TFLES) is developed, and its predictive capabilities are evaluated in an offline (or static) context. The model is then tested on the same training configuration in an online (or dynamic) context using a hybrid coupling strategy between LES and DL solvers called AVBP-DL. Coupled calculations indicated insufficient performance compared with a reference TFLES fractal model, motivating the development of a second SGS model aimed at directly predicting the unthickened LES hydrogen source term.
Jury
Prof. Alessandro PARENTE | ULB, École Polytechnique de Bruxelles | Rapporteur |
Asst. Prof. Antonio ATTILI | University of Edinburgh | Rapporteur |
Dr. Cédric MEHL | IFP Energies nouvelles | Examiner |
Prof. Annafederica URBANO | ISAE-SUPAERO | Examiner |
Dr. Stéphane RICHARD | Safran Helicopter Engines | Invited member |
Dr. Thierry POINSOT | CNRS, IMFT | Director |
Dr. Corentin LAPEYRE | Nvidia | Co-Director |
No content defined in the sidebar.