🎓Rachid EL MONTASSIR thesis defense
Tuesday 26 November 2024From 14h00 at 16h00
Salle JCA, Cerfacs, Toulouse, France
Hybrid approach based on physics and AI for probability fields advection. Application to cloud cover nowcasting.
https://youtube.com/live/aXdXZDu5kTE?feature=share
SDU2E : École doctorale ED173 “Sciences de l’Univers, de l’Environnement et de l’Espace
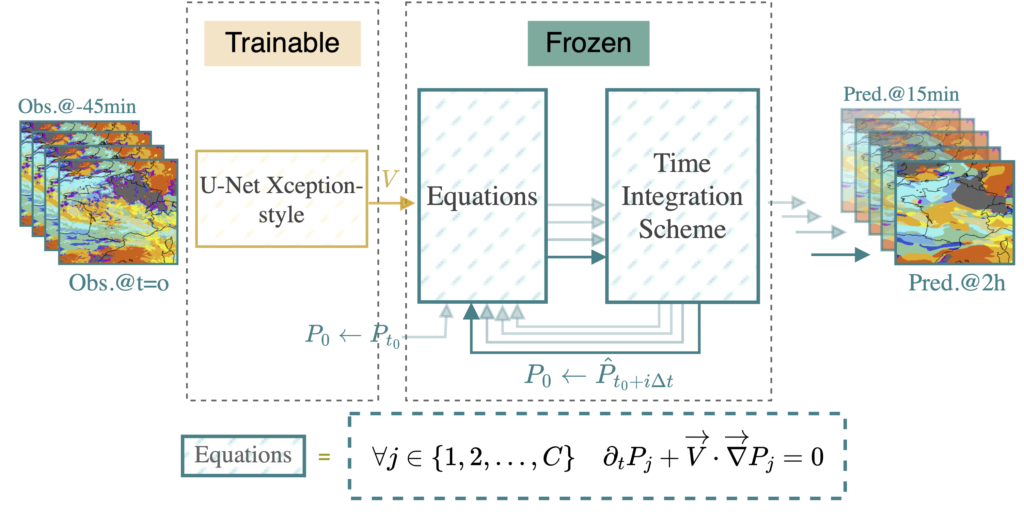
Traditional NWP models are effective but computationally expensive and struggle with small-scale predictions, while data-driven models show promise but often lack physical consistency and generalisation. This work addresses the limitations of traditional deep learning methods in producing realistic and physically consistent results that can generalise to unseen data. In this thesis, we explore hybrid methods that seek to reconcile the accuracy of first-principle methods with the data-leveraging power of learning techniques, focusing on cloud cover nowcasting, using satellite images with cloud type classification.
The proposed approach, named HyPhAICC, enforces physical behaviour based on probability advection. In the first model, denoted HyPhAICC-1, multi-level advection dynamics are used to guide the learning of a U-Net model. This is achieved by solving the advection equation for multiple probability fields, each representing a cloud type, while simultaneously learning the unknown velocity field.
Our experiments show that HyPhAICC-1 outperforms both the EUMETSAT EXIM and the U-Net in terms of the standard metrics; F1-score, CSI, and accuracy. We also demonstrate that the HyPhAICC-1 model preserves more details and produces more realistic results compared to the U-Net model. To quantitatively measure this aspect, we use a modified version of the Hausdorff distance which is, to the best of our knowledge, the first time this metric is used for this purpose in the literature. HyPhAICC-1 shows also a significant faster convergence, and performed significantly better compared to the U-Net when trained on smaller datasets, highlighting the computational efficiency of the proposed approach.
Another model, denoted HyPhAICC-2, adds a source term to the advection equation, improving accuracy but reducing visual quality.
These results suggest that this hybrid approach provides a promising solution to overcome the limitations of traditional AI methods, potentially inspiring further research to combine physical knowledge with deep learning models for better and efficient weather forecasting.
Jury
M. Fabrice GAMBOA | Professeur, IMT Toulouse III | Examinateur |
M. Guillaume BALARAC | Professeur, LEGI Grenoble | Examinateur |
M. François FLEURET | Professeur, Université de Genève | Rapporteur |
M. Guillaume GASTINEAU | Maître de conférences, Sorbonne | Rapporteur |
Mme Sidonie LEFEBVRE | Ingénieure de recherche, ONERA | Examinatrice |
M. Olivier PANNECOUCKE | CNRM/CERFACS | Directeur de thèse |
M. Corentin LAPEYRE | NVIDIA | Invité |